Researchers at the University of Michigan utilized machine learning to forecast how the compositions of metal alloys and metal oxides impact their electrical structures, paving the door for cleaner fuels and more sustainable chemical industry. Understanding how the material will operate as a mediator, or catalyst, of chemical processes, relies heavily on its electrical structure.
“We’re learning to identify the fingerprints of materials and connect them with the material’s performance,” said Bryan Goldsmith, the Dow Corning Assistant Professor of Chemical Engineering.
By analyzing the material composition of both muscle and bone in healthy patients, researchers can learn more about the mechanisms that contribute to tissue degeneration in pathological circumstances and how to cure them.
Large-scale chemical processes like hydrogen generation, the manufacture of various fuels and fertilizers, and the creation of household chemicals like dish soap might all benefit from a better understanding of which metal and metal oxide compositions are ideal for steering particular reactions.
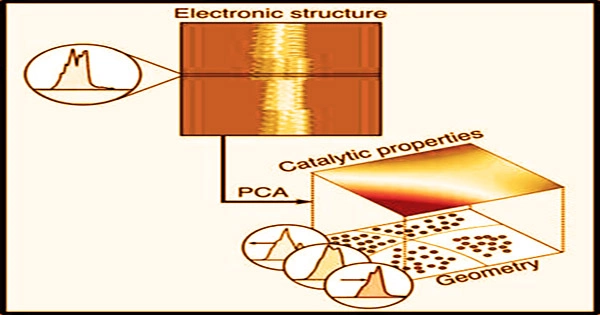
Analyzing a material’s electronic structure, especially the density of states, is one of the most used methods for predicting how it would behave as a mediator of a chemical process.
“The objective of our research is to develop predictive models that will connect the geometry of a catalyst to its performance. Such models are central for the design of new catalysts for critical chemical transformations,” said Suljo Linic, the Martin Lewis Perl Collegiate Professor of Chemical Engineering.
Product catalogs, earlier research initiatives, and available business information are used to acquire information on the material composition of each of the constituent components. If no data is provided, the material composition is calculated based on best judgment and proxy values.
Analyzing a material’s electronic structure, especially the density of states, is one of the most used methods for predicting how it would behave as a possible mediator of a chemical process. This describes how many quantum states and their energies are available to the electrons in the reacting molecules.
The electronic density of states is usually characterized using summary statistics such as average energy or skew, which shows whether more electronic states are above or below the average, and so on.
“That’s OK, but those are just simple statistics. You might miss something. With principal component analysis, you just take in everything and find what’s important. You’re not just throwing away information,” Goldsmith said.
The principal component analysis is a well-known machine learning technique that is included in beginning data science classes. Because the electrical density of states is a strong predictor of how a catalyst’s surface would adsorb, or bind with, atoms and molecules that function as reactants, they utilized it as an input for the model. The material’s composition is linked to the density of states in the model.
The team created an algorithm that they could comprehend, as opposed to traditional machine learning, which is effectively a black box that accepts data and returns predictions.
“We can see systematically what is changing in the density of states and correlate that with geometric properties of the material,” said Jacques Esterhuizen, a doctoral student in chemical engineering and first author on the paper in Chem Catalysis.
This knowledge aids chemical engineers in creating metal alloys with the desired density of states for mediating chemical reactions. The model successfully matched previously reported relationships between a material’s composition and density of states, while also revealing new prospective patterns to be investigated.
The model divides the density of states into two halves, which are referred to as major components. The fit of the metal atoms is essentially covered in one piece. This includes whether the subsurface metal is pulling the surface atoms away or pressing them together, as well as the quantity of electrons the subsurface metal contributes to bonding in a layered metal alloy.
The other component is the number of electrons that can be contributed to bonding by the surface metal atoms. They can rebuild the density of states in the material using these two main components.
This notion also applies to metal oxide reactivity. The capacity of oxygen to interact with atoms and molecules, which is related to how stable the surface oxygen is, is of significance in this scenario.
Surface oxygens that are stable are less likely to respond, whereas unstable surface oxygens are more likely to react. The oxygen stability of metal oxides and perovskites, a kind of metal oxide, was properly described by the model. The Department of Energy and the University of Michigan backed the research.